6 minute read
Modernising statistics — keeping data safe

Statistics professionals within public sector agencies take great care in how they process and protect personal data and this is reflected in the trust and respect they enjoy from their customers and the public at large. GDPR has thrown a further spotlight on governance around data confidentiality.
A significant increase in the number of new data sources and a drive to make use of administrative data means more data is being processed and published. Getting data released as close as possible to their collection date results in higher value to the economy. Transparency and open data are also driving change. There is an appetite for innovation and projects that focus on data confidentiality are happening across government.
There are two routes to get data:-
Aggregate tables are published on a website, these have had manual disclosure control techniques applied.
Access to research data is made available to those who meet specific criteria and sign legal agreements. Where data is highly sensitive the research is often conducted in a locked and secure room.
Common challenges
Data consumers
Want more data and sooner.
Want to be able to access customised tables as their needs cannot always be served by pre computed published tables.
Require more meta data to support statistical releases.
Public sector data consumers worry about a 100% push to API. They fully support open data, but are under cost pressure and do not have the resources to exploit these downstream.
Process
Myriad disclosure control techniques exist; these are manual processes.
Legal agreements to protect data access are complex and time consuming to negotiate and agree.
Data controllers want users to be able to make ‘meaningful’ queries so that data cannot be misinterpreted.
A pragmatic risk-utility balance needs be achieved and a precedent set if automation is to be successful.
Culture
Micro data access is still the norm for research purposes for a percentage of government datasets
There is no simple solution to address all of the challenges; it seems likely that a variety of different approaches will be used to address the tension between publication and statistical disclosure control (SDC).
Open source software for SDC
There are numerous tried and tested open source solutions in the market.
sdcMicro
SDCMicro is free, R-based open-source package for the generation of protected microdata for researchers and public use.
Multiple options for reducing disclosure risk and for assessing information loss.
Multiple methods for assessing the re-identification risk (k-anonymity, individual, and global re-identification risk).
Graphical user interface available for users with no or limited knowledge of the R language.
Author: Matthias Templ
τ-ARGUS
Tau-Argus is a software tool which enables statistical disclosure control to be carried out to protect tabular output. It can be run in either interactive or batch mode and can import tables or microdata, allowing the user to create tables. Tau-Argus supports either frequency or magnitude data types and once imported along with a metadata file, the user can apply a number of confidentiality rules.
Typically for magnitude tables, safety rules such as threshold and dominance rules are set by the user, and cells failing these rules are highlighted, allowing the user to select them for suppression. In order to avoid disclosure by differencing, secondary suppression can be applied using a variety of techniques. For frequency tables, controlled rounding is commonly applied. This method rounds cell values to the nearest multiple of a user specified base, whilst maintaining the table additivity.
Author: Numerous authors. Peter-Paul de Wolf — Statistics Netherlands.
SUDA
Software tool for use with statistical disclosure control for microdata. It provides a per record risk measure which not only tells the user how much risk they have but also where in the file (by record, by variable, by variable value) the risk is located. This enables the user to make principled decisions and to target disclosure control which in turn maximises the residual utility.
Author: Mark Elliot, University of Manchester
Protecting personal data with TableBuilder
TableBuilder is designed to help statistics professionals to modernise the way they process and disseminate data whilst ensuring data is kept confidential.
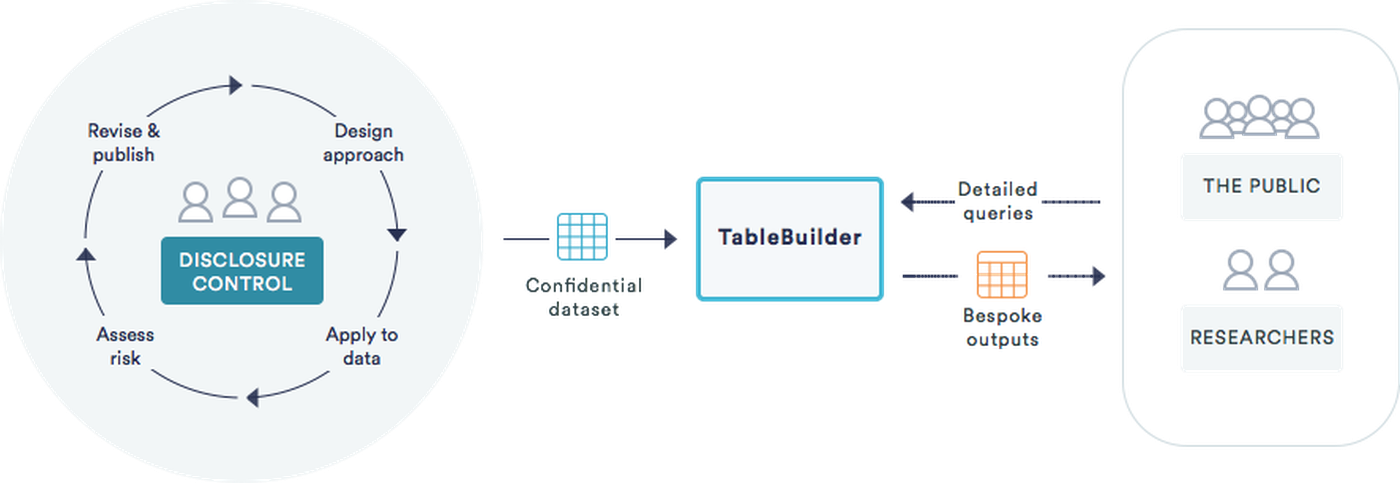
It allows flexible data dissemination through real-time application of disclosure control techniques in response to user queries.
The benefits
More granular data
More speed as data are released closer to collection data
More flexible as users can make their own queries
There is a standard user Interface and an API. Data controllers can use TableBuilder as an internal tool to model disclosure risk.
The data controller administration module allows experimentation with disclosure parameters and to see the results in real time.
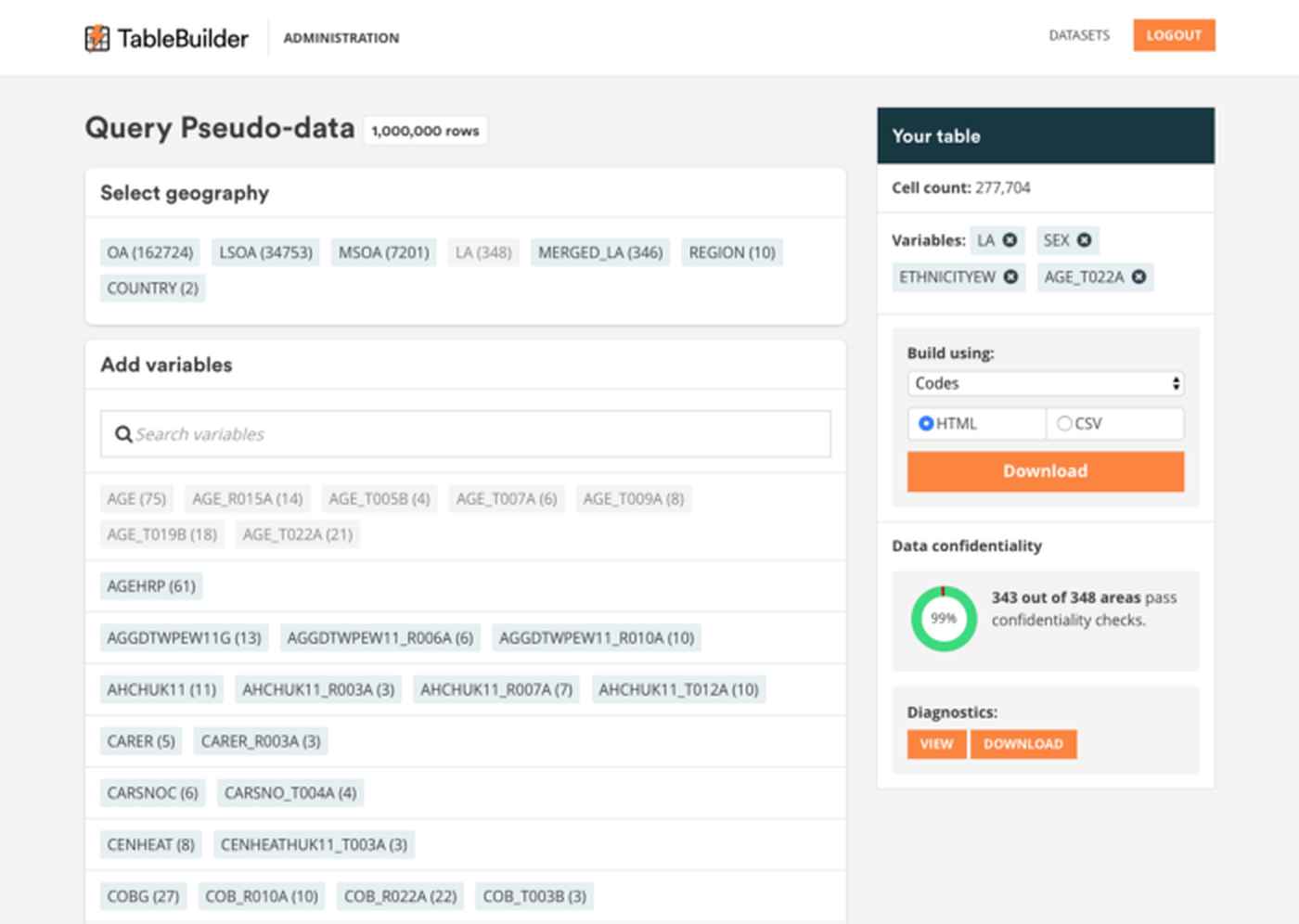
Components
Statistical disclosure control module (cell key method with automatic preservation of structural zeros)
Data controller administrator module
End user interface module
Poster presented at the International Association of Statistics in Paris in September 2018.
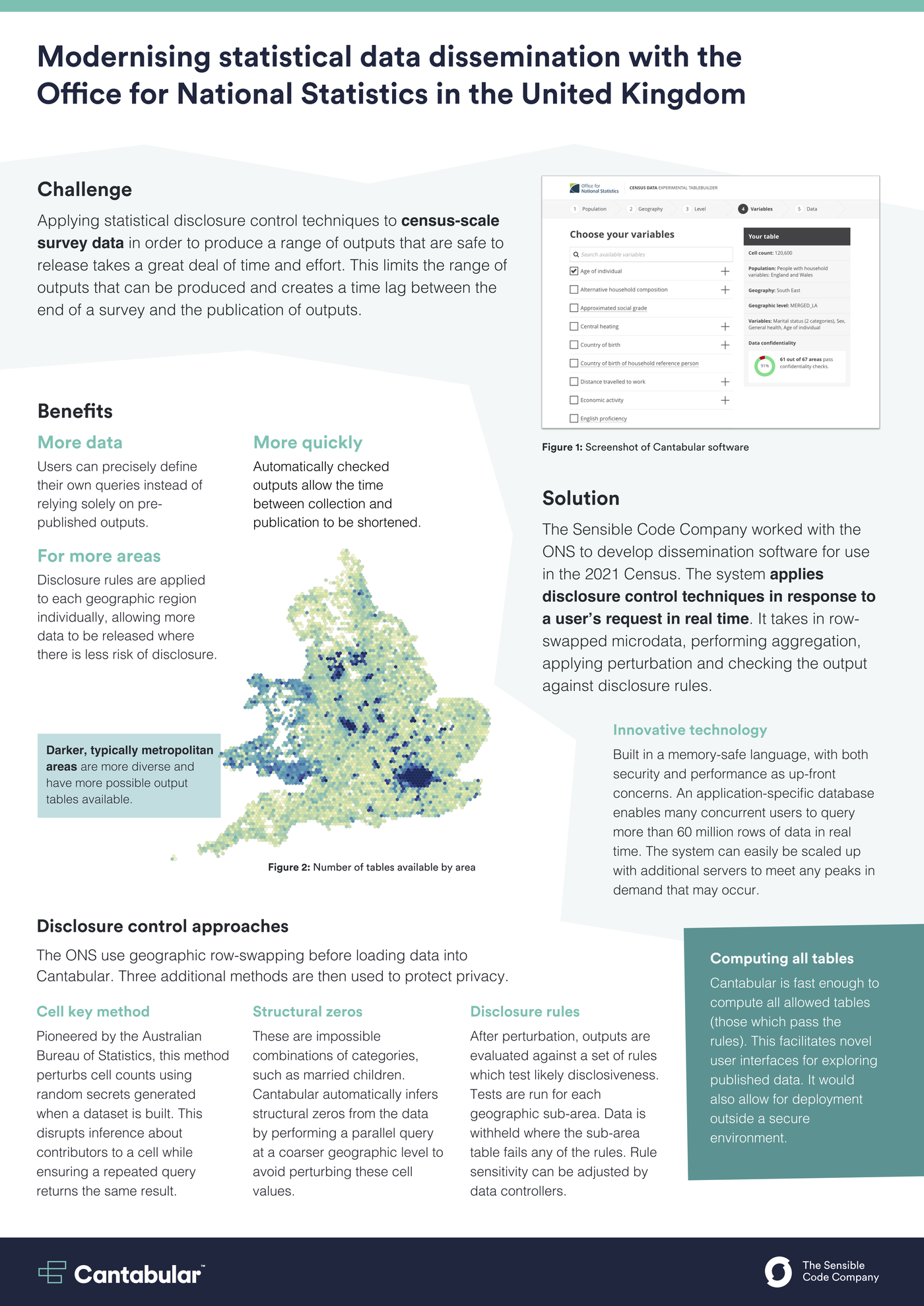